Why Healthcare Educators Should Leverage Precision Education to Optimize Learning Outcomes
In the last four decades, education has seen a significant paradigm shift, moving away from traditional instructor-led methods and toward a learner-centered approach. Healthcare education has also embraced this shift, placing learners at the center of the learning experience and promoting active engagement and self-directed learning.
In this learner-centered model, educators transition to the role of facilitators, supporting learners in developing critical thinking and problem-solving skills while fostering a lifelong commitment to learning. This approach aligns with the broader goal of preparing healthcare professionals who are adaptable and capable of meeting the evolving demands of their field.
Three Strategies You Can Adopt in Teaching to Promote Precision Learning
Download a Free Educator Guide Today
Building on this foundation, competency-based medical education (CBME) has emerged as a crucial innovation in healthcare education. It advocates transitioning from traditional time-based training to a model where learners progress based on their ability to demonstrate mastery of specific competencies such as clinical skills, critical thinking, and patient management. The emphasis is thus on performance assessment, which requires accessing, aggregating, and manipulating large volumes of performance data. CBME has been shown to help reduce medical errors, thereby enhancing the quality of healthcare and improving patient outcomes1 but the approach is not without its challenges2:
- Gaps in Competency Insufficient practical experience, inadequate feedback, and a lack of alignment between educational objectives and real-world practice may lead to some graduates struggling to provide necessary care, resulting in lower quality and inequitable patient outcomes.
- Non-Learner-Centered Assessments Existing assessment practices may not fully align with the CBME philosophy and therefore, do not accurately reflect a learner’s progress. This can lead to stress and a lack of meaningful feedback, which can hinder development.
- Variability in Assessments There is considerable variability in how assessments are conducted across different programs and institutions. This inconsistency can lead to disparities in how learners are evaluated, affecting the uniformity of competency standards and ultimately influencing the quality of care provided by future healthcare professionals.
Addressing these challenges requires a fundamentally different assessment system that ensures learners take assured, successive steps in their development—such as precision education (PE). PE shifts the focus from “how” to “why” assessment is done, and is seen as key to reshaping healthcare education to ensure equitable, high-quality patient care. In 2023, the American Medical Association (AMA) prioritized PE as one of the key drivers for advancing other high-priority initiatives in medical education.3
Precision Education: The Future of Healthcare Training
PE is defined as a system that leverages data and technology to transform lifelong learning by enhancing personalization, efficiency, and learner agency across individual, programmatic, organizational, and health system levels.4 Tailoring education to meet the specific characteristics, needs, and goals of every learner helps create a more responsive experience as learning is personalized, targeted, and therefore, more efficient. By continually refining and aligning educational experiences with the demands of the healthcare environment, PE not only enhances learning but also equips healthcare professionals to provide exceptional, inclusive care.
How does PE achieve personalized and adaptive learning experiences? It builds upon the principles of the Master Adaptive Learner (MAL) model, which emphasizes continuous development through cycles of planning, learning, assessing, and adjusting.5
The MAL model draws on several learning theories to describe how physicians and trainees not only leverage their existing knowledge but also innovate, adapt to change, and solve new problems as they arise. It makes a crucial distinction between routine expertise, where learners master skills and tasks to become highly efficient, and adaptive expertise, which allows individuals to innovate and respond creatively to new challenges in practice. Learning is considered an intentional process, where learners assess their performance, plan improvements, acquire new knowledge, and adjust their practice accordingly—a continuous cycle that equips learners with the tools they need to meet both routine and novel challenges.
PE strengthens this model by integrating data and technology to personalize learning experiences. Tools such as dashboards, which display individual-level clinical performance data relative to peers, allow trainees to assess their progress, identify areas for growth, and set goals. When paired with coaching from supervisors, this system ensures targeted feedback and real-time adjustments, supporting the ongoing cycle of learning, planning, and improvement that defines the MAL model. By focusing on both routine and adaptive expertise, PE provides actionable insights that guide more effective learning interventions.6
To better understand how PE enhances the learning experience, let’s explore the framework that supports its implementation.
The Framework for Precision Education Systems
PE offers a framework composed of four key components—inputs, insights, interventions, and outcomes—linked by four processes: analytics, planning, learning and assessing, and adjusting.
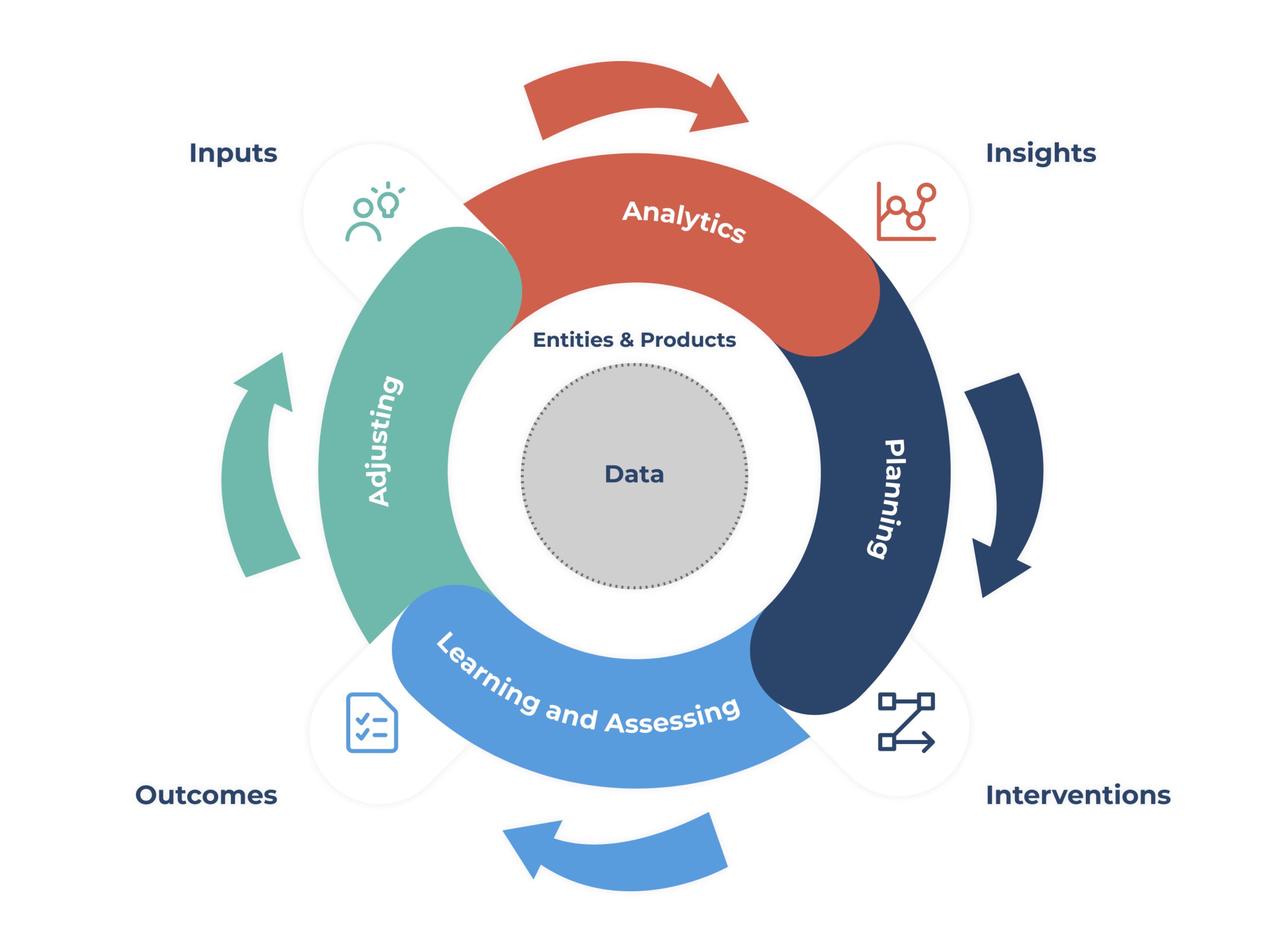
The Four Components and Processes of Precision Education
Every component and process plays a crucial role in shaping the educational process.
Inputs
What: Diverse data elements are collected from multiple sources, including assessment, educational activities, electronic medical records, patient outcomes, and clinical practices.
Why it’s important: Provide essential data to inform the educational process at various levels—individual, programmatic, and organizational.
Insights
What: Inputs, when fed through advanced analytics, transform raw data into actionable insights.
Why it’s important: Help identify gaps or achievements in knowledge, skills, and experiences, guiding the development of personalized educational interventions.
Interventions
What: Guided by insights, these can range from just-in-time educational content to long-term strategies addressing specific educational needs and are tailored to individual learners or groups.
Why it’s important: Help drive the planning and implementation of personalized learning, efficiently addressing the specific needs of individual learners through targeted support.
Outcomes
What: Measure the effectiveness of the interventions at various levels—individual, program, and organization.
Why it’s important: Outcomes are continuously assessed and fed back into the system to refine and improve future educational cycles.
Focusing on the four components allows PE to create a more personalized, efficient, and impactful learning environment while the cyclical process ensures that it remains adaptive, continually evolving to meet the needs of learners and healthcare systems—ultimately enhancing educational and patient care outcomes. In healthcare education, PE is further shaped by the 4Ps framework of precision medicine: predictive, preventive, personalized, and participative.7 Just as precision medicine tailors care to individual patients, PE customizes learning pathways to meet each learner’s unique needs, ensuring timely and efficient interventions.
One crucial element lies at the heart of this continuous cycle of adaptation and improvement: data.
The Importance of Data in Precision Education
Data is essential in PE; aggregated data provides insights into curricular effectiveness, identifies areas for improvement, and supports interprofessional collaboration by highlighting gaps and opportunities across healthcare disciplines. This enables educators to tailor learning plans that meet each learner’s specific needs, not only ensuring targeted support but also real-time feedback, helping learners achieve competency more efficiently.
Data Integration: 3 Levels of Interconnectedness
The three levels of PE—micro, meso, and macro—are interconnected, with data from each level informing decisions and improvements across the system.
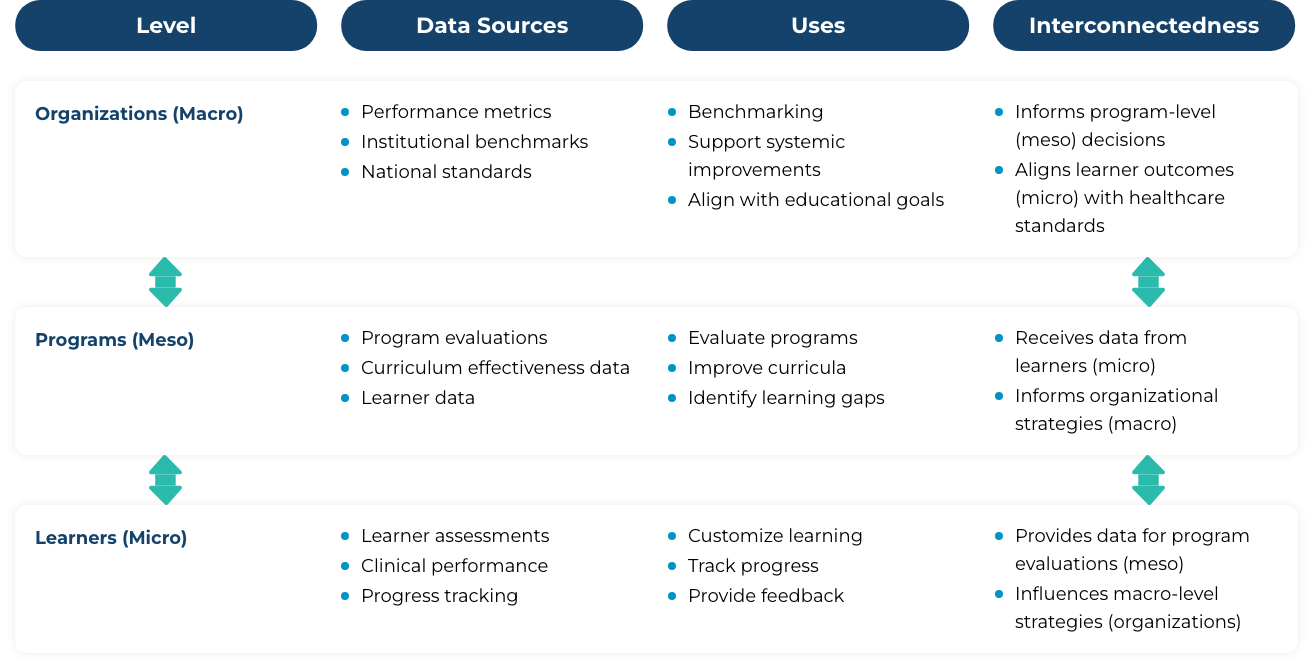
For such a comprehensive application of data to work, a unified data system is key. Fragmented and inconsistent data structures across the medical education and healthcare ecosystems must be unified before PE can reach its full potential.
The Way Forward for Healthcare Education
Establishing PE systems is a complex matter that is not without its challenges, but worth the effort as it has shown to have tremendous potential to transform healthcare education. Learners more easily develop higher-order thinking skills and lifelong habits that empower them to become adaptable healthcare professionals capable of delivering high-quality patient care.
Programs like the Graduate Medical Education Laboratory (GEL) and the Transition to Residency Advantage (TRA) have demonstrated how a data-driven, personalized education can better meet the needs of learners and institutions—signaling that PE is the future of healthcare education.8
References
1 Bhattacharya S. Competency-based medical education: An overview. Annals of Medical Science & Research. 2023;2(3):132-138. doi:10.4103/amsr.amsr_27_23.
2 Schumacher DJ, Santen SA, Pugh CM, Burk-Rafel J. Foreword: The next era of assessment and precision education. Acad Med. 2024;99(4S):S1. doi:10.1097/ACM.0000000000005609
3 American Medical Association. Precision education. American Medical Association. Published July 2, 2024. Accessed September 2, 2024. https://www.ama-assn.org/education/changemeded-initiative/precision-education
4 Desai SV, Burk-Rafel J, Lomis KD, et al. Precision education: The future of lifelong learning in medicine. Acad Med. 2024;99(4S Suppl 1). doi:10.1097/ACM.0000000000005601
5 Cutrer WB, Miller B, Pusic MV, et al. Fostering the development of master adaptive learners: A conceptual model to guide skill acquisition in medical education. Acad Med. 2017;92(1):70-75. doi:10.1097/acm.0000000000001323
6 Drake CB, Heery LM, Burk-Rafel J, Triola MM, Sartori DJ. A theoretical foundation to inform the implementation of precision education and assessment. Acad Med. 2024;99(4S):S30. doi:10.1097/ACM.0000000000005604
7 Baiardini I, Heffler E. The patient-centered decision system as per the 4Ps of precision medicine. In: Implementing Precision Medicine in Best Practices of Chronic Airway Diseases. Elsevier; 2019:147-151.
6 Garibaldi BT, Hollon MM, Woodworth GE, Winkel AF, Desai SV. Navigating the landscape of precision education: Insights from on-the-ground initiatives. Acad Med. 2024;99(4S):S71. doi:10.1097/ACM.0000000000005606